
AI Disrupts Itself, Expect More of This
DeepSeek echoes a popular pattern in technology: the incumbent building bigger and more expensive tech, only to be disrupted by someone more clever.
Besides all the societal disruptions AI has caused, AI has disrupted itself. The new DeepSeek R1 model roughly matches the performance of the previous leaders but differs in multiple dimensions: Chinese vs. American, open source vs. proprietary, state-of-the-art hardware vs. almost state-of-the-art hardware, and an order-of-magnitude difference in the training cost. In an industry using brute force to power its way to the front, the nimbler competitor training smarter rather than harder is the best. While the DeepSeek researchers have been publishing papers about their methods for years, the robust capacity of the model surprised the world and caused a fair bit of hysteria. Many investors had bet that proprietary models would dominate the market, trained on mass amounts of expensive, specialized hardware, and the market quickly reflected their loss. However, for society, the emergence of a powerful, relatively inexpensive open source model allays the concerns about companies with proprietary LLMs dominating the market and using their power to stifle smaller competitors, limit access to generative AI models, and charge monopoly prices.
This echoes a popular pattern in technology: the incumbent building bigger and more expensive tech, only to be disrupted by someone more clever. For example, Yahoo had an enormous platform built on powerful and expensive servers, constantly being upgraded to more powerful versions. Many saw their lead as impenetrable because of the enormous cost of acquiring comparable hardware to compile the vast indices for a search engine. Then Google swooped in with both a superior algorithm and the ability to use less specialized hardware, both of which were easier to scale. Switching costs for search engines are quite low, like those for generative AI models, and Yahoo quickly lost its dominance. The replacement of large and expensive mainframes with the personal computer is similar.
The R1 advantage is mainly a function of the leanness of its training process. Instead of explicitly programmed rules, it uses a reinforcement learning technique to guide itself and be more autodidactic. This rewards it for reaching desirable end states rather than telling it exactly how to get there. Rather than one large, monolithic model, DeepSeek uses a Mixture of Experts architecture, in which an ensemble of specialized models each train on a subset of the data, learning that subject area in great detail. When a user queries the model, a router sends it to the right expert. Intuitively, using a monolithic base model is like relying on the smartest person in the room to answer every question. By contrast, the Mixture of Experts architecture is analogous to directing queries to a panel of specialists, directing a query to a doctor, mechanic, librarian, etc., as appropriate. This is more efficient in training and query answering processes, handling more complex problems with improved accuracy and less training data. Proprietary models ( e.g. OpenAI’s ChatGPT-4) also employ Mixture of Experts architecture. However, DeepSeek is claiming that they spent orders of magnitude less money training their model. DeepSeek used cheaper hardware, but the widely reported $6 million cost only reflects pre-training costs, excluding the costs of research, testing, salaries, and running the hardware. When these are added in, the total tops $100 million. It’s likely cheaper, but not drastically. However, it follows a pattern that building on existing tech is usually faster and more efficient. Knowing where previous efforts failed can help companies avoid development dead ends, leaping over those with a first mover disadvantage.
DeepSeek’s R1 model is certainly not the first open source model to perform relatively well, but others have not quite measured up to the proprietary models on industry standard benchmarks. While there is nothing inherently superior about proprietary models, they are very expensive to build because of the costs of acquiring training data, buying the specialized hardware and computing power to train them, and salaries for the highly skilled engineers involved. Companies tend not to want to share something they invested so much money in. However, the proprietary vs. open source gap has been closing over the past two years, and it is not surprising that an open source model is the current leader. A vast community of researchers and software developers, including many academics, enthusiastically contribute to open source – not just improving the model’s performance but shining a light on bugs, code quality issues, and security vulnerabilities. They are from diverse backgrounds, enriching software with their particular expertise, e.g., expanding language support. The community's contribution and review of the code foster trust in the model. Additionally, open source models can be customized and extended for additional domains, use cases, and model behaviors. This avoids the technical and legal barriers to improving and extending proprietary models.
For users, accessing R1 to answer queries is significantly less expensive than using its proprietary competitors, although their prices have fallen drastically in the last year. Users can also host their copy without paying for the model, just the hardware to do inference to answer the incoming queries with R1. The reduced price allows smaller organizations to access the model, including individuals, startups, non-profits, and academics. It’s hard to foresee the full effects of this increased accessibility to cutting-edge models on society. However, in previous cases where open source software has matched proprietary technologies, it has fueled massive innovation and empowered entire ecosystems. Open source software such as Linux (Unix operating system), PostgresSQL (relational database management system), Python (programming language), and TensorFlow (machine model framework) have spawned massive ecosystems because they are more adaptable and transparent and, thus, can be extended more easily. Additionally, open source technologies often lead to voluntary industry standards, increasing interoperability and accelerating technological development.
DeepSeek is a Chinese company, causing concerns that the Chinese government will have access to user queries. While this is likely true for the particular copy of the model hosted by DeepSeek, users can host their own, perhaps even on “air gapped” hardware not connected to the internet. Users explicitly enter queries into the tool even for the hosted version, avoiding tacit background data surveillance associated with phone applications. Users may not think about their privacy when they ask, but they know their data is being collected (as it is in American models). However, as people tend to put their innermost and most private thoughts into their search engine queries, this will likely not stop them. Also, there are likely to be biases (read censorship) in R1’s answers. Proprietary American models also have these types of filters, e.g. censoring answers that would be racist or lead to inexpert medical advice. One should be aware when using any model that biases (and hallucinations) may distort the answers.
R1 is an open source algorithm, which means that DeepSeek’s competitors can build on it, too. In a month, or perhaps sooner, another company will likely release an open source model that duplicates this feat and probably exceeds its accuracy and efficiency. The media will probably have a panic attack about that, too, although probably less so if it’s not Chinese.
Rachel Lomasky is Chief Data Scientist at Flux, a company that helps organizations do responsible AI. She has helped scientists train and productionalize their machine learning algorithms throughout her career.
Economic Dynamism

The American Dream Is Not a Coin Flip, and Wages Have Not Stagnated
This paper challenges the prevailing narrative that stagnant wages are causing the American dream to fade. It contrasts subjective public opinion with revised objective intergenerational mobility measures.
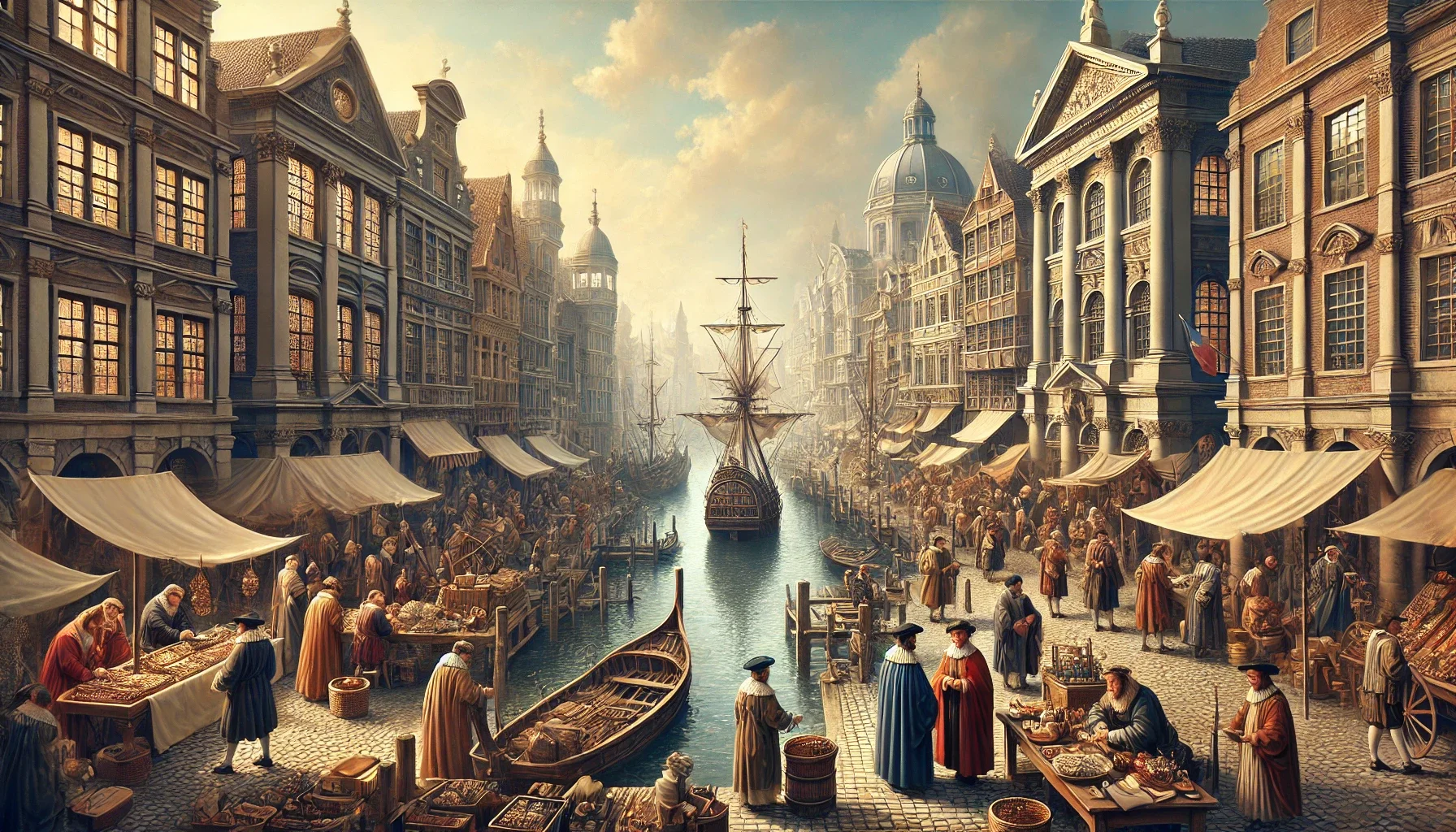
Political Economy and the Rise of Commercial Humanism
Western attitudes toward commerce have transformed from early moral condemnation to a modern appreciation that sees trade as socially beneficial.
.webp)
A Bad Business on the Bayou
Chevron finds itself the victim of a political alliance between the tort bar and Louisiana Republicans.
.webp)
Congress Must Shield US Companies from European Regulations
Congress should exercise its constitutional powers over foreign commerce to guard American companies against overregulation by the European Union.
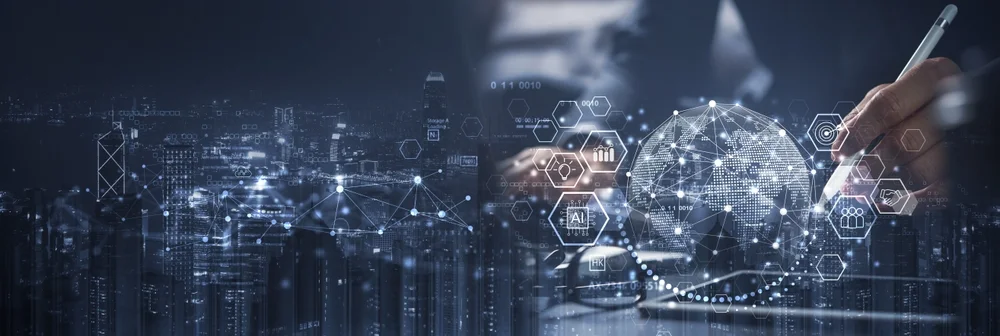
Trump Undermines His Growth Agenda for AI
An innovative ecosystem relies on market-driven capital formation, fast scaling, and wide and ready access to inputs—all of which are undermined by the policies many Republicans now embrace.

There Is No Substitute for Free Trade and Deregulated Markets
The world economy will teeter so long as the U.S. wields its tariff stick.