.webp)
Why the AI Revolution Will Require Massive Energy Resources
Training large-scale models requires enormous computation, fueled by multimodal datasets comprising text, audio, video, images, and sensor data that often exceed a petabyte.
The rapid rise of generative AI has triggered a sharp escalation in data center electricity consumption, with profound implications for national energy use, system planning, and climate goals. Data centers have long been critical infrastructure for digital services, but their energy demand is now accelerating due to the emergence of compute-intensive AI workloads.
Data center electricity use began climbing after plateauing around 60 terawatt-hours (TWh) annually from 2014 to 2016—roughly 1.5 percent of total U.S. electricity consumption. By 2018, it had reached 76 TWh (1.9 percent of national demand), driven by growing server installations and an architectural shift toward AI-optimized hardware, particularly Graphics Processing Units (GPUs). This upward trend has since intensified. By 2023, U.S. data center electricity consumption had surged to an estimated 176 TWh, representing 4.4 percent of total U.S. demand, roughly equivalent to the annual electricity use of the entire state of New York.
This growth shows no signs of slowing. The U.S. Department of Energy projects that by 2028, annual electricity demand from data centers could reach between 325 TWh and 580 TWh, or 6.7 percent to 12 percent of projected national consumption. Forecasts from firms such as Boston Consulting Group and S&P Global similarly place 2030 data center electricity use between 5 percent and 10 percent of total U.S. demand. The range of estimates reflects uncertainty in how quickly AI technologies will be adopted and how widely compute-intensive applications will scale.
At the heart of this demand surge is generative AI. Training large-scale models requires enormous computation, fueled by multimodal datasets comprising text, audio, video, images, and sensor data that often exceed a petabyte. While data acquisition and storage carry energy costs, the training process itself is far more energy-intensive, as it depends on the model's size, the complexity of its architecture, and the degree of refinement. Training is a one-time event per model but demands vast amounts of power, time, and hardware resources.
After training, models are used for inference, generating outputs in response to user queries. Each inference consumes far less energy than training, but because these systems are queried millions of times daily, their cumulative energy use becomes substantial. More complex outputs, such as videos or high-resolution images, increase the burden.
Generative AI workloads depend heavily on specialized chip architecture: (GPUs) and Tensor Processing Units (TPUs). These chips are optimized for the matrix operations at the core of AI computation. While they are more efficient than general-purpose CPUs for such tasks, they also draw significantly more power and generate more heat. As a result, they require constant and often intensive cooling, which in turn demands additional electricity and, in many cases, fresh water. Marginal improvements in chip design, such as more compact transistor layouts and power-aware software, have improved performance per watt. Similarly, advances in cooling that range from more efficient fans and heatsinks to liquid cooling and immersion systems help reduce waste heat. However, these innovations have not yet offset the exponential growth in demand.
One promising way to mitigate energy use is to reduce the computational intensity of the algorithms themselves. Smaller, specialized models can be trained with less data, lower numerical precision, and fewer iterations, making them faster and less costly. Techniques like transfer learning, where a pre-trained model is adapted for a new task, and federated learning, where training is distributed across edge devices rather than centralized, can also conserve energy and reduce data transfer loads.
Still, overall energy demand continues to rise—a textbook example of the Jevons Paradox, where efficiency gains lower costs but stimulate greater total consumption. Yet generative AI may also produce net energy savings in other sectors. For example, dynamic routing algorithms can optimize delivery truck routes based on real-time traffic and weather data, reducing fuel use. Similar gains are possible in building HVAC control, precision agriculture, and industrial automation. Thus, while AI’s direct energy footprint is growing rapidly, its broader potential to improve energy efficiency while increasing economic productivity may partially offset these impacts.
Lynne Kiesling is Director of the Institute for Regulatory Law & Economics at Northwestern Pritzker's Center on Law, Business, and Economics; Research Professor at the University of Colorado, Denver.
Rachel Lomasky is Chief Data Scientist at Flux, a company that helps organizations do responsible AI.
Economic Dynamism

The American Dream Is Not a Coin Flip, and Wages Have Not Stagnated
This paper challenges the prevailing narrative that stagnant wages are causing the American dream to fade. It contrasts subjective public opinion with revised objective intergenerational mobility measures.
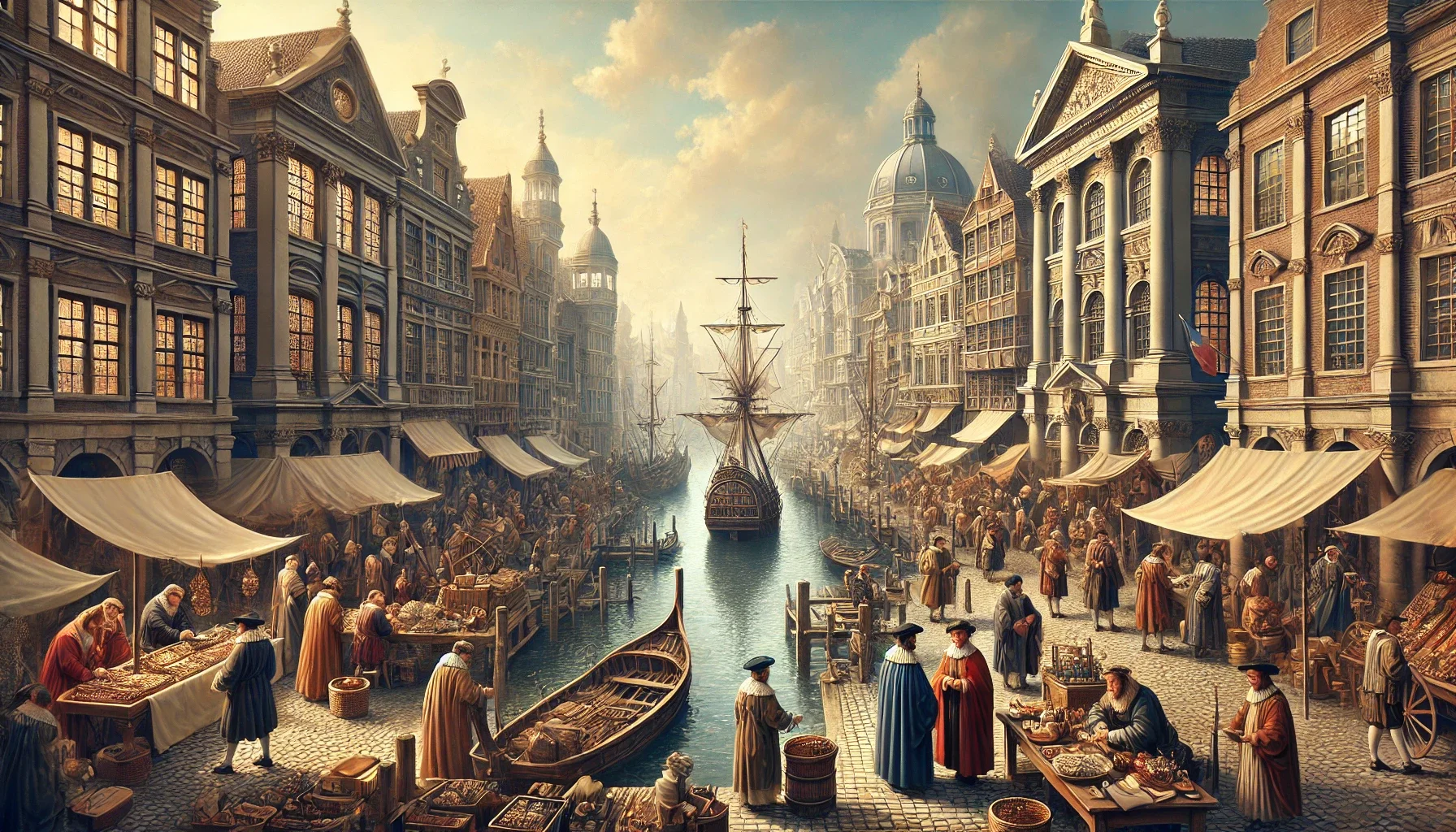
Political Economy and the Rise of Commercial Humanism
Western attitudes toward commerce have transformed from early moral condemnation to a modern appreciation that sees trade as socially beneficial.
.webp)
A Bad Business on the Bayou
Chevron finds itself the victim of a political alliance between the tort bar and Louisiana Republicans.
.webp)
Congress Must Shield US Companies from European Regulations
Congress should exercise its constitutional powers over foreign commerce to guard American companies against overregulation by the European Union.
.png)
Improving Commerce and Security in the Americas: A Civitas Outlook Symposium
What possible ways forward might bring peace, commerce, and flourishing?

Enhancing Trade, Security, and Peace in the Americas: The Role of Think Tanks
The victory of Argentinean Javier Milei, an advocate of the free economy and national defense and an opponent of the 2030 agenda, has encouraged many like-minded groups not to give up on Latin America.