
The Right and Wrong Way to Regulate AI
The regulatory frameworks seem desirable on the surface but become idealistic, contradictory, and impractical upon further examination.
The proliferation of AI systems, particularly generative AI, has led to a public demand for increased regulation. Some of the worries are hysteria, such as the claim that AI poses an existential risk without discussing a potential mechanism for such risk. Yet, to trust it enough, the public must be assured that reasonable concerns about biased and incompetent AI systems, particularly for high-risk use cases, can be managed well. Additionally, while people often state desires for strict AI regulation, their revealed preferences show that most freely share their data in exchange for convenience and rarely check AI outputs for correctness. But the problem remains that regulation could endanger AI's future growth and promise of improving multiple aspects of human well-being. The very nature of AI development and system application makes it difficult for the tools of regulation to monitor it and enforce rules against its activities. In many cases, doing so would dramatically slow, if not render impossible, the AI industry from achieving new breakthroughs. Thus, an effective framework will provide guardrails around dangerous systems while allowing the market to innovate responsibly. A reasonable solution allows flexibility for the variety of AI system types, use cases, degrees of autonomy, social cost of malfunction, etc. Additionally, it must accommodate individual preferences about trade-offs in dimensions like privacy, convenience, system performance, and price.
There are several proposed frameworks for ensuring AI is ethical, effective, and secure, ranging from voluntary recommendations to punitive regulations. They share broadly overlapping tenets, generally grouped together under the label “Responsible AI” (RAI). The underlying tenants behind RAI are orthogonal to the system type and apply to AI, automated, human, and hybrid processes. For example, if there are guardrails to keep a pilot from making a mistake, we should ensure that an autopilot doesn’t make the same mistake. The burden should be on the organization proposing additional regulations to prove why the copious existing regulations are insufficient.
The frameworks seem desirable on the surface but become idealistic, contradictory, and impractical upon further examination. They focus almost exclusively on the (necessary) task of ensuring AI does not act poorly and very little on ensuring that AI remains open to innovation and development. Usually, they are significantly more demanding than equivalent controls on corresponding human processes. Thus, as a whole, they have a dampening effect on AI experimentation, particularly in the investment that attracts investment and accelerates development.
Across the RAI frameworks, demands that the systems avoid biases for protected characteristics, such as race and gender, are the most common. Remediating biases and other performance issues in the case of prediction accuracy might be prohibitively expensive or impossible. Biases in AI systems are usually a product of underlying societal biases in the input data used to train the algorithms as the result of systematic biases, e.g., differences in consumption of healthcare in different populations. AI regulation will not remediate them. Not all biases are unjust, and some are even desirable. Not all biases are unjust, and some are even desirable. We want the AI system disproportionately to recommend health care for older adults. Additionally, reducing bias can also reduce model quality, as an AI system designed to approve loan applications must use input variables that correlate with income, which then correlate with other sensitive demographic variables, such as age. Not considering income would decrease model quality, but it would be best to avoid gender bias. This is just one example of how a superficial RAI statement becomes more nuanced with further investigation.
Many RAI regulations require the ability to understand and attribute blame for violations. However, determining accountability for AI failings can be difficult, particularly when several individuals and organizations are in the complex workflow of collecting data, training and testing the algorithms, implementing the use cases, monitoring the performance and bias, and remediating issues. Thus, rationally, there is a call for transparency in how the AI makes decisions, particularly when it is autonomous. RAI tenets ask that outputs be understood as a function of the importance of the input variables, and ideally, inputs can be tracked step-by-step through the algorithm until outputs. Understanding how decisions are made can lead to greater trust and, ultimately, better system performance because it allows troubleshooting and improving pitfalls. However, sophisticated, highly performing models tend to be very difficult to interpret in this manner, resulting in difficulty in understanding the root cause of bias or poor performance. A successful framework must treat explainability as an ideal and allow the users to balance a preference for a comprehensive understanding with a desire for a more accurate model.
A large number of RAI rules apply to automated systems, regardless of whether they use AI. For example, systems should be robust to unexpected inputs and secure from attacks, including on the underlying data. Similarly, consent should be granted on gathered data, kept private, and only stored if necessary. Monitoring should be used to evaluate system performance and adherence to ethical guidelines. Additionally, systems within acceptable limits of performance and bias can fall out of compliance without deliberate changes by practitioners or users of the system, particularly for systems based on foundational generative AI models, e.g., Gemini and ChatGPT, which are outside the users’ control of the people building on them. In addition, models that adapt in response to data can be sabotaged by malicious behavior or disruptive events, e.g., covid and natural disasters. Even with self-contained, static models that receive scheduled upgrades, predicting whether performance will suddenly decline, or predictions will become biased can be difficult. Previously, libraries and services remained stable. Because of these technical challenges, practitioners are responsible for monitoring their applications, but there must be realistic expectations about time to remediate the transgressions.
Several guidelines call for users to be notified and sometimes consent to automated decisions. While it may be valuable for a user to know the quality and biases of the systems, it is irrelevant whether the results came from an AI, human, or hybrid process. This requirement signals to non-technical people that they should have increased skepticism about automated processes despite them being much easier to audit, fix, and monitor than humans. It also leads to “alarm fatigue,” people so overwhelmed by the notifications that they don’t read and, therefore, miss the important ones. The label raises anxiety about AI, hindering its spread, similar to how the GMO label retarded the spread of inexpensive and more environmentally friendly food. In some cases, e.g., when ethical decisions must be made, humans should review or even make the final decisions. However, this should not be universally true.
Many governments seem to believe that they can enact strict regulations to force responsible AI practices. However, punitive AI regulations in one jurisdiction will cause companies to relocate to more lightly regulated environments or fail completely. For example, venture capital investment in Europe is lagging far behind the Americas and Asia, partially due to the enormous costs of the GDPR privacy legislation and a fear of the upcoming EU AI Act. If there is an overwhelming regulatory burden on AI, some companies will withdraw their services, particularly the smaller companies currently providing AI, which don’t have the resources to create multiple versions of their products for varied regulations. Of course, bad actors will completely ignore the regulations, and it will be hard to identify them and ensure that they do not keep morphing and reentering the services ecosystem.
Additionally, the opaqueness of AI models can make regulatory enforcement difficult. Understanding model bias and performance requires a thorough analysis of the organization’s proprietary data and technology, something they would be loath to share. Even once a regulator evaluates a system, a model can become non-compliant without action by the organization, e.g., if the foundational models shift or there is concept drift in the data. Fixing the issues may take significant time and effort, causing either a rogue model to remain accessible or be taken offline, causing a service disruption. Even if a model meets regulatory standards, it may still be used for an unethical use case. For example, the same facial recognition algorithms can help parents find their lost children or for the state to identify political enemies.
Thus, the ideal is an agile framework that protects against unethical AI practices while encouraging robust experimentation. It allows intelligent decisions about which AI systems they use without assuming a huge investigation burden. People can roughly understand an AI system's principles without thoroughly evaluating system details. Certifications are time-limited and elastic because of the dynamic nature of AI technologies, particularly generative AI. Thus, people and organizations choose systems that meet their risk tolerances for a given application and ethical considerations for the particular use case.
Meeting these requirements with a regulatory framework would be impossible because it would ignore individuals’ varied weightings for privacy, convenience, and price for AI/Big Data-based service, as well as the technical limitations in achieving an understanding of the underpinnings. On the other hand, without guidelines, individuals would be overwhelmed by the amount of research necessary to understand an AI application, and therefore, this is also untenable. Informed consent would require the consumer to invest significant time for each system to understand all of the components of model training, including how the data is being gathered and stored, model training, and the strengths and weaknesses of the model for their particular case. Users need to understand the organization and usage behind the system. For example, some users might consent to their anonymized medical data being leveraged by a nonprofit fighting a disease but would be upset if the data were used commercially.
The experimental nature of AI systems requires a set of voluntary, flexible, and adaptable frameworks offered by a private certification and licensing regime managed by industry experts sufficiently knowledgeable to develop realistic and innovative regulations and standards. There is a well-established pattern of Industry Self-Regulatory Organizations (ISROs) in tech being responsive to emergent issues; for example, ISROs adopted IT certifications for the Internet of Things and cybersecurity certifications for new threats.
Certification agencies are motivated to ensure that their rules are sufficient for ethical and accurate models but not too stifling. Agencies can offer multiple levels of certification with varied ethical principles, performance standards, auditing, and monitoring. Organizations can choose which one to pursue to match their values and needs, e.g., depending on security, privacy tradeoffs, and market demand. While users may have ideals for their AI systems, they may compromise in the face of other considerations, including price and convenience. For example, some people may be willing to use a chatbot that is occasionally racist if the performance is otherwise excellent. For example, for highly sensitive use cases, they would choose a level that included investigating the underlying technology, more frequent audits, etc. But for low-risk systems, a quick and easy process may be sufficient, perhaps even conducted by AI examining the code with minimal human intervention.
As a result, organizations would feel more comfortable experimenting with innovative technologies without fear of fines and other penalties from government regulatory regimes. For systems that pose a low risk to public safety, this will encourage rampant experimentation while providing suitable guardrails around those that are more dangerous. As AI is nascent and rapidly evolving, thus making it difficult to codify collective choices, this level of flexibility is necessary. If the system is sensitive, it is already covered by regulation and, potentially, by industry standards. This approach balances the need for innovation with the imperative for safety, allowing for responsible AI development while minimizing experimentation hurdles.
Rachel Lomasky is Chief Data Scientist at Flux, a company that helps organizations do responsible AI.
Economic Dynamism

The American Dream Is Not a Coin Flip, and Wages Have Not Stagnated
This paper challenges the prevailing narrative that stagnant wages are causing the American dream to fade. It contrasts subjective public opinion with revised objective intergenerational mobility measures.
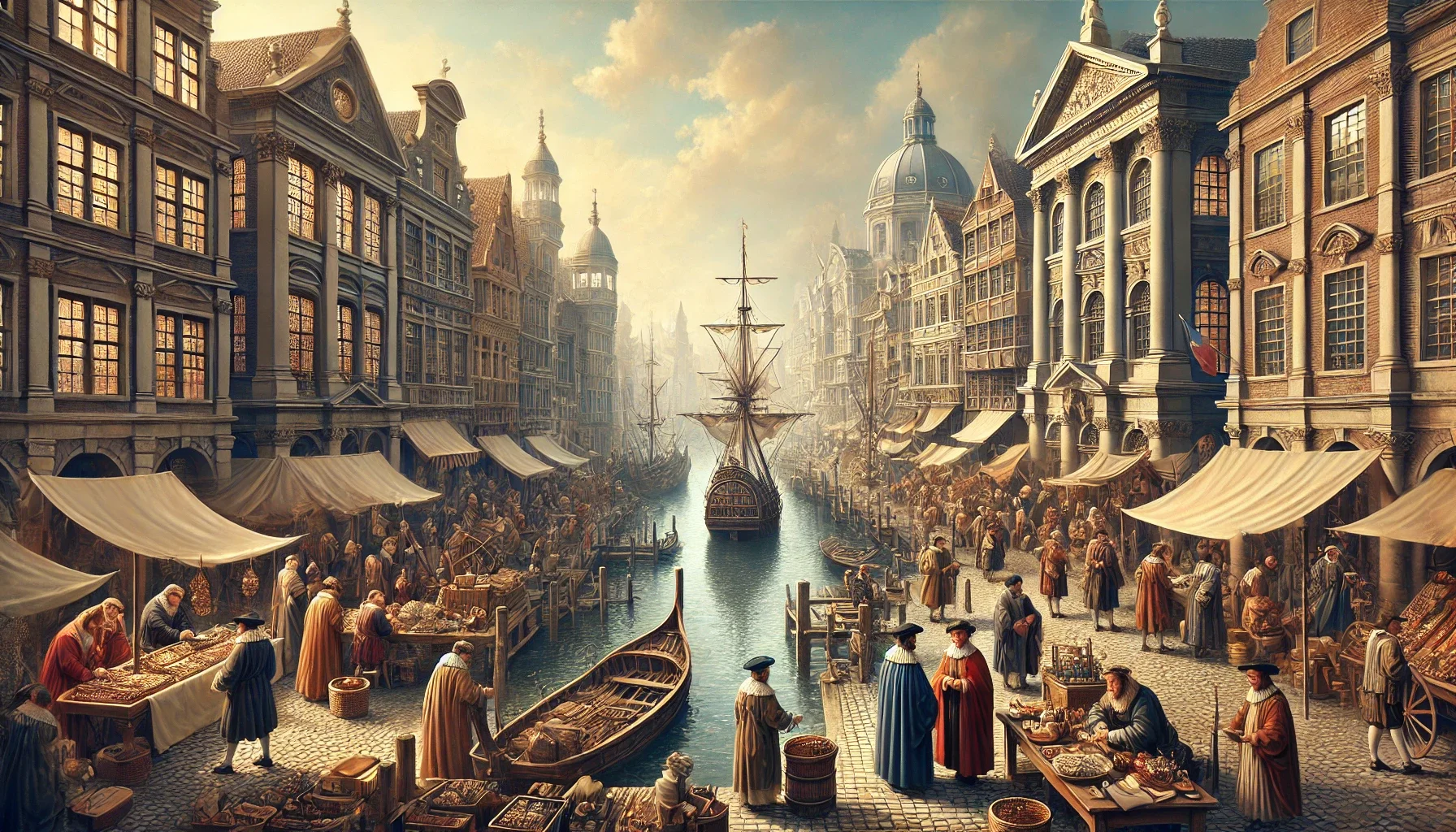
Political Economy and the Rise of Commercial Humanism
Western attitudes toward commerce have transformed from early moral condemnation to a modern appreciation that sees trade as socially beneficial.
.webp)
A Bad Business on the Bayou
Chevron finds itself the victim of a political alliance between the tort bar and Louisiana Republicans.
.webp)
Congress Must Shield US Companies from European Regulations
Congress should exercise its constitutional powers over foreign commerce to guard American companies against overregulation by the European Union.

Trade Protection and Tariffs from Reagan to Trump
Donald Trump's enthusiastic embrace of tariffs and protection for American industry appears to be a complete reversal of Reagan and Reagan-era policy.

Transatlantic Trade War Produces No Winners
The EU should end its competition policy vendetta. Trump should drop his tariffs.